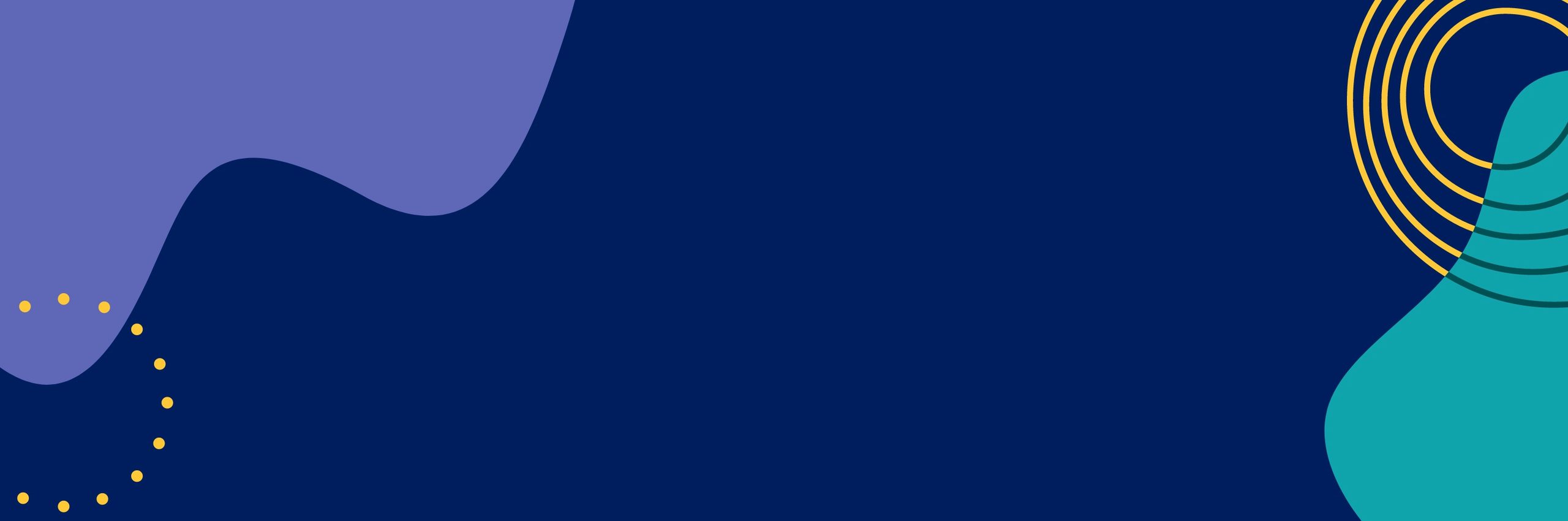
Predictive analytics

What is predictive analytics?
Predictive analytics combines historical data, machine learning algorithms, and statistical techniques to forecast future customer behaviors and trends. Predictive analytics predict customer needs, preferences, and actions – so businesses can create more personalized, effective marketing and customer engagement strategies.

Why is predictive analytics important?
With predictive analytics, businesses can deepen their understanding of customers, anticipate their needs, and engage them more effectively. It all adds up to higher satisfaction, loyalty, and revenue.
Benefits of predictive analytics
- Predictive analytics determine the most effective next best action to engage a customer – whether that’s sending a personalized offer, following up on a service issue, or taking another relevant step.
- Predictive analytics estimate the customer lifetime value, or future value a customer will bring to the business over their entire relationship. This informs business decisions about how much to invest in marketing activities.
- Predictive analytics can identify customers who are at risk of churning based on their interaction patterns, purchase history, and engagement metrics. This allows companies to take proactive measures to retain at-risk customers.


How does predictive analytics work?
Predictive analytics takes steps involving data collection, data processing, model building, and implementation within AI-powered technology solutions. Businesses will walk away with increased efficacy in their marketing and customer engagement programs.
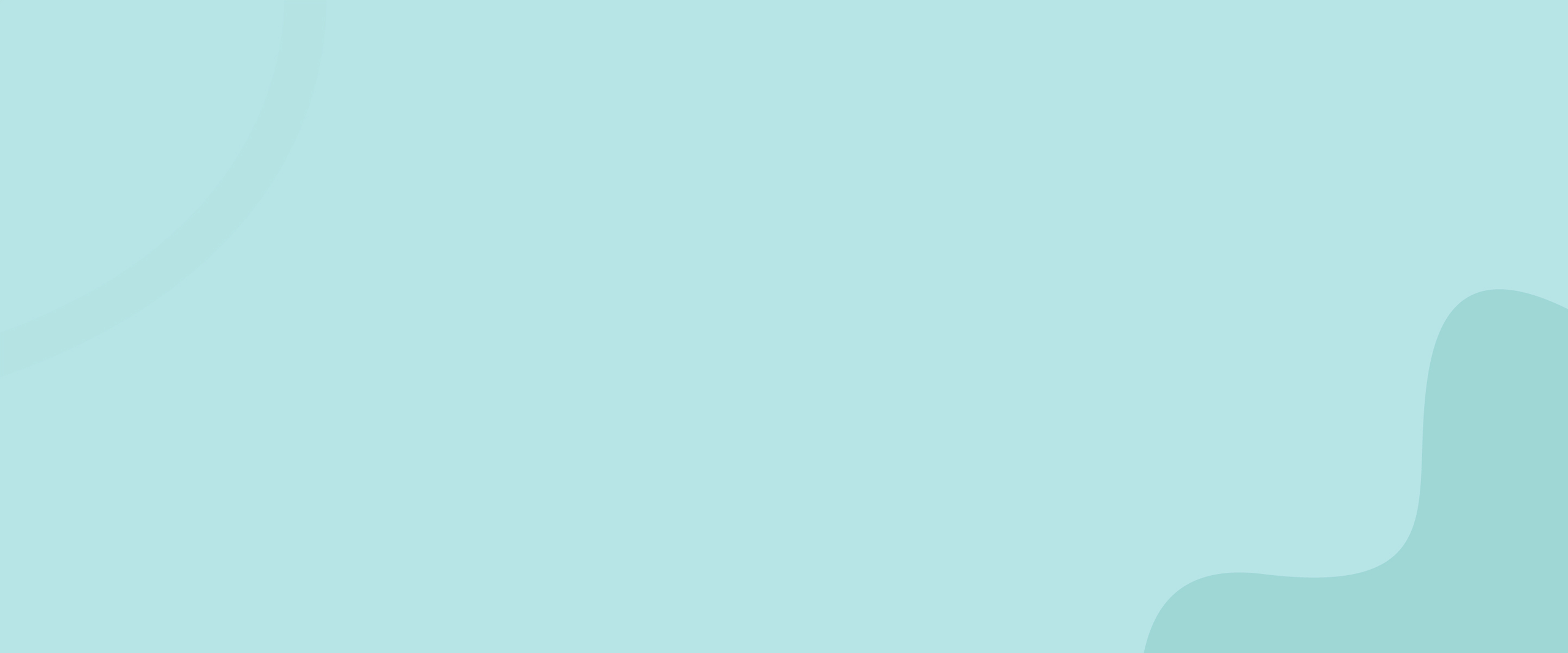
Product
Deepen relationships and maximize value with real-time decisioning.
Common techniques used in predictive analytics
Data collection
Collecting data from various sources that aggregate transaction, behavioral, demographic, interaction, or customer feedback data.
AI-powered predictions
Using machine learning models and algorithms to predict future behaviors based on patterns in historical data sources.
Customer engagement integrations
Embedding predictive models into customer engagement platforms like CRM systems, marketing automation tools, and other marketing technology solutions.

Challenges of predictive analytics
Utilizing predictive analytics does pose challenges, however businesses can address and mitigate them proactively.
- Data quality and integration can be challenging – with data from various sources being stored in different systems or departments, creating silos. Not to mention data may have inconsistencies, missing values, or inaccuracies, complicating the creation of reliable predictive models.
- Some machine learning models, especially complex ones, can be difficult to interpret. That makes it challenging to understand how decisions are made, which may introduce or amplify AI bias.
- Predictive analytics relies primarily on stale historical data that lacks context versus real-time data, making it difficult to provide the most relevant and timely customer experience possible.
- Building and maintaining predictive analytics models requires specialized skills in data science, machine learning, and domain expertise. When budgets are constrained, allocating sufficient resources for these initiatives can be challenging.
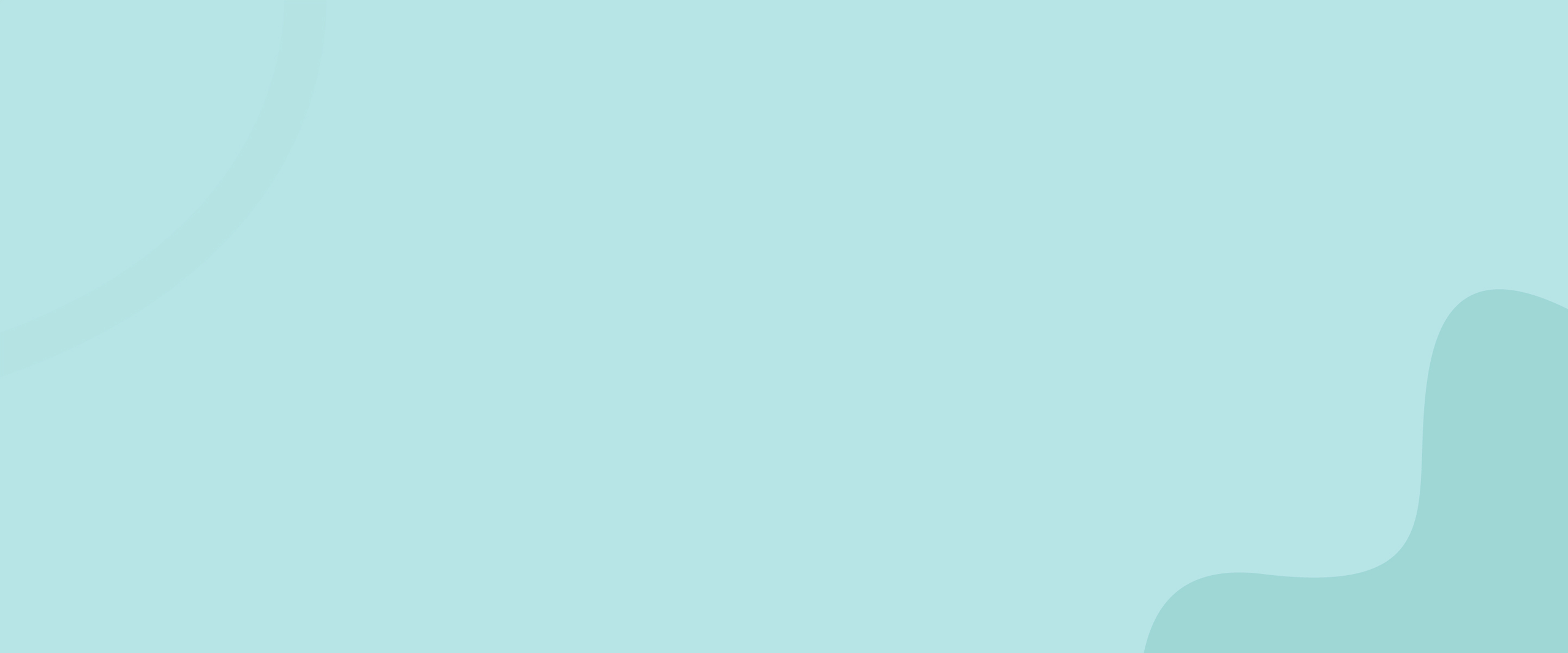
Technology
Pega’s next best action works to make every interaction more relevant and meaningful.
What are some use cases for predictive analytics?
Customer journey mapping
Predictive analytics can map out potential customer journeys, identifying key touchpoints and predicting customer actions at each stage – allowing businesses to optimize the customer experience.
Cross-sell and upsell opportunities
By analyzing purchase history and customer profiles, predictive models can identify opportunities for cross-selling and upselling, suggesting complementary products or premium versions of existing products.
Campaign and program optimization
Predictive models can forecast the performance of different channels, programs and campaigns, helping marketers allocate budgets more effectively and achieve better ROI.
How to get started with predictive analytics
Get started with predictive analytics by defining your objectives and goals, collecting and preparing data assets, identifying how to measure success, and designating subject matter experts to implement effective technologies and programs to best achieve improved customer engagement and business outcomes.

Frequently Asked Questions on predictive analytics
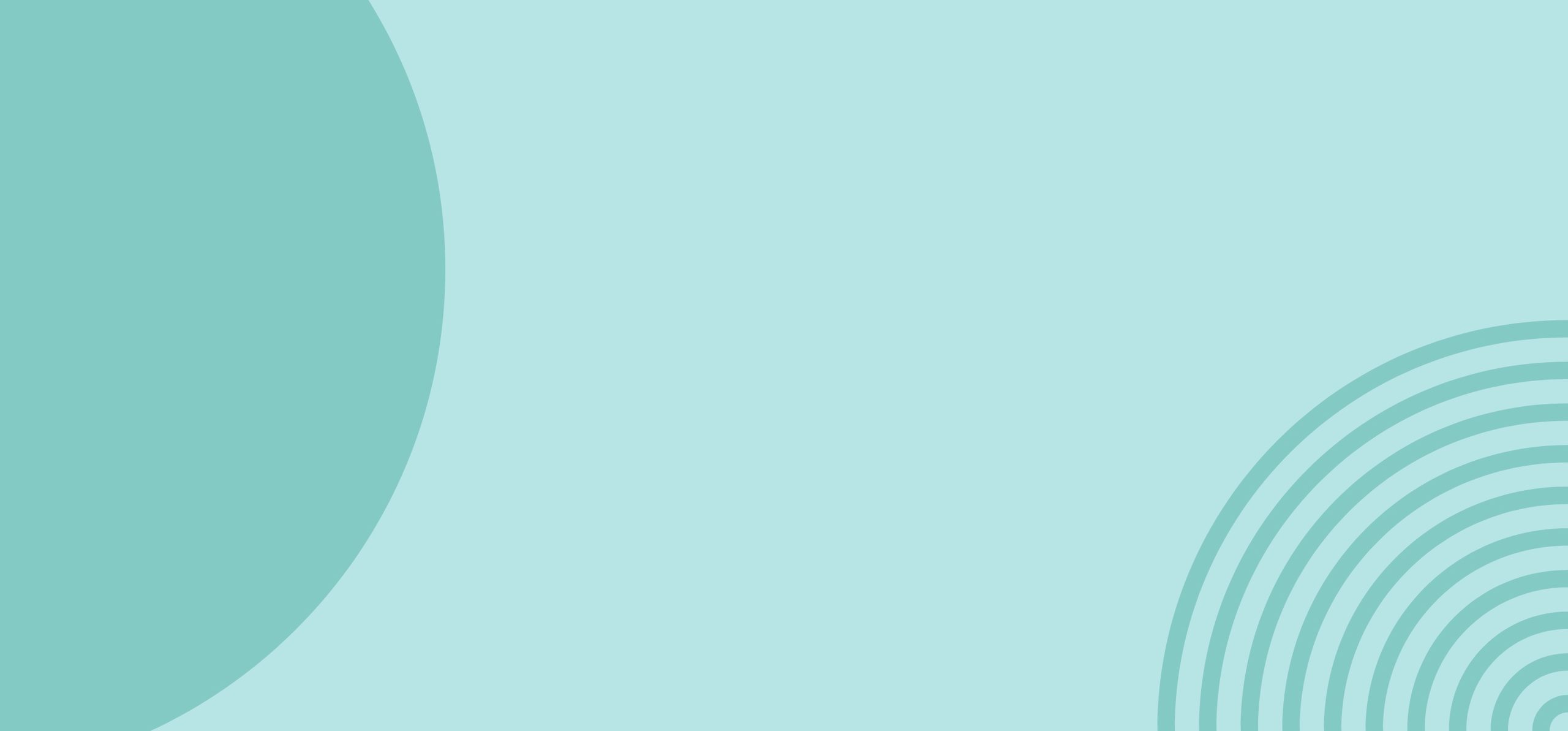