PegaWorld | 46:10
PegaWorld iNspire 2023: Mapping the AI Landscape: From Generative, Predictive to Prescriptive AI
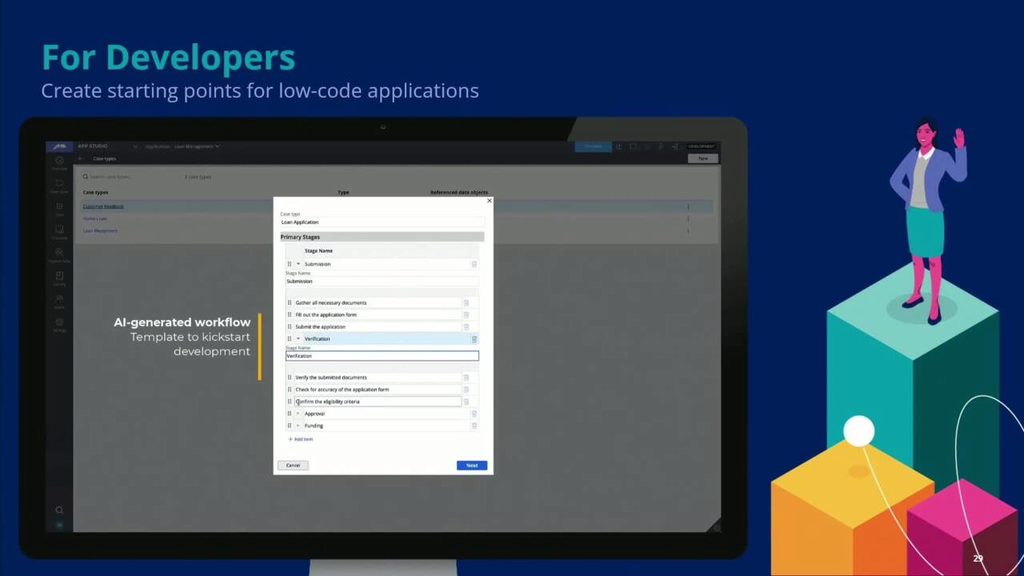
Get ready to explore the world of AI and unlock its potential business applications with Pega. From Google searches to AI-generated playlists on Spotify, we are surrounded by artificial intelligence every day. Now, with generative AI systems like ChatGPT, we can take an even more hands-on approach. But have you ever thought about how the creative power of generative AI can be combined with Pega's intelligence and workflow capabilities to revolutionize your business? Watch this session to see how!
Transcript:
Peter van der Putten:
Thanks for coming out to this breakout here on mapping the AI landscape. My name is Peter van der Putten. I'm the director of Pega's AI Lab. So I look at how businesses can transform their business using AI, but we also apply it to Pega itself. So developing new AI-led go-to markets like Process AI, and of course the most recent thing, which is GenAI. So I helped kick that off ... incept that. And in our lab, we continuously monitor what's going on in AI and see ... try out these new technologies and see how we can provide more value to our customers.
Today I'll be joined here with two of my colleagues, who are really working in the area of making AI real, really turning it into a product. Shoel from product management and Christian in engineering. So they built the real products that we are going to be talking about here. And of course there was no shortage of attention for AI and specifically for GenAI here at this conference. It was hard to find a keynote where it wasn't really being talked about. And if you would go to our website today, you can see it's actually front and centre on the website, "Generative AI, now for the enterprise." So what I'll do, I'll run maybe a quick video just to refresh ... do a very quick refresh on the various ways how we're using AI in our platform nowadays.
So if you ... Well, that's a lot of capabilities, of course, over 20 boosters that we announced across our platform and applications. And if you think like, oh, that went really quick, don't worry. In the rest of the breakout, we'll go into detail. I'll talk a little bit more about how do large language models work in the first place. Christian is going to talk more about how do we architect for this? What are some of the principles? And Shoel will take us through some of those boosters and use cases. But I thought it would be nice also to talk a little bit about how did we actually get here. And for that, I'm going to talk about something which is a personal project of mine. So it's not even a Pega project, but I think it's a nice illustration of how the creative powers of foundation models or large language models can be used to drive change outside of enterprise use cases.
And there's a bit of a background story to this because GPT-3 came out in May 2020. And already, around July 2020, I got access to GPT-3.5. That's a year and a half before it became publicly available. And because I had been working with generative models already for a couple of years, but I really saw that 3.5 was the big step change. So I was lucky enough ... you could see a little tweet to the CTO of OpenAI that got me that access. And then a friend of mine came to me, he's an AI artist. He said, "I have an idea about a project where we might be using ... might have a good use for AI." And his project was all around climate change. And he said, "Well, we have all kinds of things in nature that under threat of climate change, but nature doesn't have a voice. So could we use something artificial like AI to help nature by giving it literally a voice?" So that was the general idea.
So we generated all kinds of letters from sinking islands and melting glaciers and drying up deserts to world leaders like the Pope, et cetera. And then we found different ways to communicate these messages to the outside world. So you see here a museum in Germany, ZKM, and we had a movie running on the outside of the museum with all these letters and messages from these natural elements inside. Also, we had a little movie, but we want to keep it simple. So we had all these letters just stamped to the wall, and we were hoping that people will actually get lost in the work as they were exploring it and starting to read all these AI-generated letters. And fortunately that was really happening. And then we found different ways to communicate the outcome. So for example, a big projection here on the museum on the side of the museum in the Netherlands. And as you can see, the AI gets quite creative. You would think AI is something hard and cold, but it's really a technology that we could use here to communicate a lot of emotional expression.
Now, why am I showing you this? I think it is a good example ... when we go back to business, but it is a good example of how AI is entering into the creative realm. And we've been using AI a lot for rational left-brain type of AI, optimal decision-making. But a generative AI adds creativity on top. So to position that a little bit more, last year, it was already early mid-last year that we decided to go all in with generative AI, way before ChatGPT came out. You see some articles I contributed to the Wall Street Journal. And we then decided we want to layer generative AI on top of the AI capabilities we already had. Because you can see here that we had quite a few AI capabilities around NLP or process AI, voice AI, machine learning, predictive analytics, real-time decisioning. And we're layering the generative AI actually on top of that. Or a different way to think about it is, like I said, we can go from predictive AI, more to prescriptive AI, real-time decisioning, and now generative AI is feeding creativity into that process.
Or just to give you the third metaphor and more the metaphor of left-brain and right-brain. So the left-brain, we could do planning, sensing, predicting, deciding, memorizing, learning, all the rational aspects. But right brain of course would give us this creative capabilities of generative AI. And if I paint a conceptual picture, ultimately what AI is being used for here is then sensing the environment. So when you're dealing with a customer, it could be all kinds of things that are happening around that customer, maybe a claim that comes in and circumstances. And then we make smart decisions on what to do next. Can we approve this claim or should we route it to a claims agent, for example? And then we take that particular action, we measure the outcome, and we learn. So it's a big cybernetic feedback loop that we're essentially implementing here where generative AI is actually plugging into that sense, the site and the loop.
And the key question you may have is how do these large language models actually work? Well, they're trained on billions and billions of tokens, hundreds of billions of tokens, characters actually more or less. Actually one token is three to four characters. And the people that build these generative AI models, they black out these tokens or characters in the text, and then they ask the AI to predict what is the missing text here? And the funny thing is by doing that basic task, but using huge amounts of data on very large models, it needs to start to develop an understanding for syntax, for semantics, how to disambiguate certain words, et cetera. So it's learning all kinds of general language skills. If we then do a little bit of additional training on particular tasks like summarization, et cetera, then you'll have a model that's able to carry out all kinds of tasks, even tasks it wasn't really trained for.
And what we see, of course, that whole generative AI landscape, it's truly exploding. Where there was two years, three years actually between GPT-3.5 and ChatGPT for example. After that, there was ... over a course of time, you can see there's an explosion ... can be an explosion of these foundation models and large language models. And that creates opportunities but also it's a little bit worrisome because how can you develop all kinds of new use cases if in the background that ecosystem is still developing so quickly? And that's not the only concern that we need to deal with. You also need to think about many different risks, like the system could actually hallucinate. How do we deal with company-sensitive data? How do we check for bias? How do we govern this all? And I wouldn't be talking about problems here if there was no solution. And that's essentially Pega GenAI, which is a future-proof enterprise architecture for trustworthy generative AI. And I would like to ask Christian to talk more about the principles and how we architected for this.
Christian Guttmann:
Great, thank you. Yes, thanks for the introduction. And yeah, my name is Christian Guttmann. Thank you. VP of Engineering Decisioning and AI. And I will share a few insights as to how we build all this and how all these demos and use cases you have seen today are actually what's under the hood, so to say. So let's see if I press the right one. Yeah. So the way we built these capabilities, we are guided. We have essentially an approach. We have certain guidelines by which we follow when we build our platform. One of them is that we want to build a future-proof architecture, which we have pretty much always done as a company. And that means that we don't want our clients to be locked in for certain GenAI capabilities. So obviously as you have heard earlier, there are many providers of these LLMs, these language models, and our platform actually ... we want you to choose and to be able to have basically access to many of them.
Another one is that we have a unified API governance model, meaning that we have a centralized way of overlooking questions on licensing, monitoring and controlling those different APIs. So we have pretty much everything centralized and we have an overview and an understanding of how all these functionalities work. And maybe another one is human-in-the-loop. You have heard it a couple of times by Karim and also Don in the keynote speeches. We want to make sure that whatever is generated is also reviewed by a human at any time. So these are some examples of how we are making our GenAI work. So now I'm going a little bit into detail. So that's our approach, our let's say overview of an architecture that we have been building out. Our teams at Pega have been working on many of these components that you see and many of these boxes that you see are available in Infinity 23.
I won't be going through all of them, but just to give you an idea. On the top left corner you see the generative AI use cases that Karim mentioned earlier and that Shoel will essentially go through in much more detail. But the connect generative AI rule that you see, which is a second box from the top on the left, that is essentially a way how you can interact with the GenAI capabilities, prompt engineering being one of them. You probably are familiar that the way you ask large language models to do certain tasks is very important and that prompt engineering box allows you to do that in a very good way. So there's a lot of nifty engineering that goes into that. And the same also with the response engineering. So how do you want that data to be given back and being entered back into the system basically? So that's an important aspect of this particular rule.
It's pretty much also the front end. It's what you're seeing in the platform in CDH or in the other apps and products that we offer. On the bottom, the foundational layer, that's pretty much the back end. What can I highlight here? For example, the privacy data filtering. So here we have a functionality which allows to replace and to ensure that private and confidential data does not leave your organization. And so there's also GenAI way of doing that. I highlight one more example, which is the AI model gateway, which I believe Karim also touched on earlier in the presentation today. And that's basically allowing you to choose ... or rather, well, there's a choice. Either you can choose which models to use or our system helps you to choose the best possible models that are applicable for your use case.
And that's this yellow box in the middle on the right. And basically those boxes that are orange that are all on the right, they are running in the Cloud. And one of the reasons is that the Cloud services that we build have these capabilities ... have much more capabilities for us to expand on the GenAI use cases basically. I just make a quick mentioning on the top right autopilot box. I think that's actually a very exciting area. It turns out that's actually an area that I've been working with for the last ... yeah, also, as Rob said, too long to maybe mention on stage, but that's the area that I've been working with a lot. And I think it's super exciting because you can give that system the ability to perform autonomously a whole lot of very complex tasks. So that's actually very exciting area for us to go into. Okay, and then, just to summarize, pretty much-
Shoel Perelman:
Maybe you want to call out the lower right-hand corner also, what we're doing with our own local models also.
Christian Guttmann:
Yes, I can do that. Hang on. Can I go back?
Speaker 4:
Yeah. Right there. This one.
Shoel Perelman:
There you go.
Christian Guttmann:
Yeah. I'll try it. Here we go. Too much. Okay. Do you mean the bottom right one?
Shoel Perelman:
Yeah. The lower one.
Christian Guttmann:
With our own models? Yeah. So I mean, and that's also what Karim mentioned earlier today where he saw this big box and in that big box on the right corner where the internal models that we are offering in our platform basically. And they are much more specific to the task. So for example, summarization there, the summarizer model, these are models that do not require necessarily outside calls, but they are very functional for a specific purpose in this box. And so they're internal to our platform.
Shoel Perelman:
Yeah. And I just thought I would ... I'll add to that. The reason why we've done that is because in conversations ... and of course nobody's had more than a few months of conversations because we've all started getting excited about this mostly in November of last year when ChatGPT came out. But one of the topics that comes up a lot is data privacy. And so Christian was talking about that capability that will help you filter out PII data from making its way through to a model.
Now some clients, they have a enterprise agreement with Microsoft and they have no problem sending PII, right? But others may have ... everybody's going to have their own security policies that you're surely going to go through as an enterprise. And so we felt it was important for us also to be able to provide a way for executing generative AI running entirely within the confines of our Pega Cloud managed environment. Of course, for specific tasks. So as Christian was saying, there are the general purpose ones, but for something like summarization, which I'll talk about in a little while, there are very specialized models that start from being open source that we can fine tune. And we wanted to provide the ability for running those entirely within Pega Cloud also.
Christian Guttmann:
Yep, well said. And of course we can go into details, so if you have questions on that slide or the model, then please approach us afterwards.
Shoel Perelman:
Yeah. Start thinking of your questions. We're going to try to blast through this early and save 10 minutes for ... at least 10 minutes for questions.
Christian Guttmann:
Correct. Correct. Okay. So summarizing this slide, really the generative AI connect rule type. So as I mentioned before, these are really product capabilities that we have been and we are building out that will be accessible in '23. I mentioned some before. We have a simple way of prompting. We have a prompt interface, dynamic contextual inputs, the ability to mask and unmask PII, as mentioned earlier. So there's a whole lot of functionality around that. And yeah, also the ability to return structured and unstructured responses. Basically they can be turning directly into data types, as we have seen earlier today and yesterday too. Or in unstructured responses, which would be like free text, for example. So I think-
Shoel Perelman:
And I'll just add some context. So if you're in the audience and you are typically building Pega applications yourself, you will become very familiar with this connect generative AI rule because that's the way we're exposing generative AI in the Pega platform model. If on the other hand you are using one of the applications that we provide on top of platform like CDH, you'll be insulated from this connect generative AI rule. We've used it to build our own application and all the infrastructure that Christian was talking about is underneath the connect generative AI rule.
Christian Guttmann:
Excellent. And then that's also good lead way for you to take over and expand and go deeper.
Shoel Perelman:
Happy to.
Christian Guttmann:
There you go.
Shoel Perelman:
So let's talk through some of the use cases. We have a couple of categories of use cases, but going to be, as we talked about, right now, if you're an application developer, there're going to be the ability for you to build this into your applications. As Christian was talking about, we've built what we call response engineering into that rule. What that really means is if you guys have all played with ChatGPT, if you ask it to generate something in JSON for example, you'll get something back. What we've done is we've done the work to parse what comes back and make it available back on the clipboard for you to use in the rest of your application. Customer engagement, these are all the examples that are ... So I'm the product VP for CDH, for Customer Decision Hub, so I can speak in depth with some special love about those. But customer service is right next to CDH, right? It's the other side, the part that is about empowering humans to have interactions with your customers. And there's a tremendous opportunity for generative AI to help those humans do a better job.
And then of course, back end, this is going to be largely about what you build into your apps, but also helping you query those apps and better understand what's happening behind the scenes. So let's go into a couple of them. This is something that ... we recognize that you guys are going to go back, you're going to fly home from here, and you will often find yourself in the room having to convince others in your company why a certain use case is a great fit for Pega. Right? We recognize that that happens and we love you for the fact that you do that. But we recognize that sometimes your constituents need to be able to see it with their own eyes in order to get it. So we're actually going to be building a way of being able to prototype out quickly what a case might look like.
If you're just sitting down with a business stakeholder, you ask them what the name of the case is, describe it, you'll be able to go to a website, type it in, and you'll get a quick rundown of what that might look like, all the stages and steps directly in your browser. That's of course for prototyping, for brainstorming. But we then have the continuity of being able to do that same thing in the actual product. So you can type that same case name in, and this is the video that you saw earlier, where we'll go through and it will, in a more structured way, create the case, create all the stages, create all the steps. Obviously, you'll need to tweak them, right? Because this is not just a demo at this point. You'll be creating real infrastructure that will run for your enterprise.
One of the things that I like best about that, by the way, I know we've skipped forward, is the data model. So as I've been playing with it, I've been fascinated ... at first it was like a parlor trick, but then I got to the realization that it's actually very good at this, is you can just take a use case and you could ask it to design the data model for you. And it usually is pretty good. And the reason why I'm so excited about that is having been involved in many projects, I've realized that it's not so much the technical work, it's the fact that you may have to spend two, three weeks in a conference room with many people getting them to agree on what should be in that data model, as opposed to you can generate a pretty good starting point and let them critique it on their own time and you could update it from there. So I actually think that's going to be a huge time saver.
What we're showing here is robotics. So robotics came from an acquisition we did a couple of years ago and it has a tremendous number of use cases here, like being able to validate credit cards. Or another one I think is validate that a phone number is valid for a given country. Pretty hard thing to do if you ever try to write a regexp for validating a phone number, you need to have knowledge of each country. But because generative AI has been trained up on the knowledge of the internet, it's actually pretty good at doing these things just right out of the box. And it will generate the code for you. So this is another one I'm very excited about. This is ... we call this self-study buddy. You can use this yourself, but we recognize this pattern. We have an internal chat room within Pega with hundreds of people, and often we'll have consultants saying, "How do I do this certain thing?"
And as product people and developers, they'll sometimes think, "RTFM, it's in the manual." And so somebody within the company got very inspired by this problem. And so they thought, you know what? I bet generative AI could do a pretty good job at answering many of these questions that get asked. And so what self-study buddy will do is if you ask it a question about Pega platform, how something works, it can be CDH, customer service, anything you want, it will scour through the documentation, find the factual answers, not hallucinated answers, and give you an answer to your question, even if it requires looking at a few different documents and stitching together the answer. So I think this is actually going to lead to some of the very exciting opportunities once we start marrying that with the developer experience, that you're in the application, you're trying to build something, you need help and advice on how to do it, it will be able to actually guide you straight in the application. That's what Alan was referring to in his keynote yesterday.
This is one that I thought was ... by the way, a lot of these came out of ... like many of you, you've probably had hackathons, right? So we had our own hackathon back in March. And so we had about 300 different use cases that came out of that. So the 20 that you're seeing here and that you saw written up in our press release, we call them boosters, those were the best of the best that came out of that hackathon. And this was one of the winners. So for those who may be familiar with using Pega for customer service, you'll know that we've had natural language processing capabilities in the platform for about seven or eight years. And the good thing is it's battle hardened, right? You can train it up, it will not hallucinate, it will give the answers that you've approved. But the downside to that is if you ask the question in not quite the right way with the right terms, it won't give you an answer.
So this idea is blend these together. If we don't know the answer to something, if our internal NLP hasn't been trained on the answer, ask GPT, "What are five different ways of saying the same thing asking this question in a different way?" Try that out against the NLP to get the answer. And once we find the answer, now we can train the NLP to recognize these five other ways of asking that same question. And this applies also for voice AI, where you never know how a human is going to ask a question. So I think it's a great example of blending these two worlds together. Here's another one in the customer service space and it is I think quite creative. It is using GPT to train up your agents. So you have new CSRs that are coming on board and you want to role play with them.
So the GPT will play the role of an angry, cranky customer asking them curve ball questions and they have to figure out what answers they should give or even do they know what buttons to click on in the UI, in the customer service UI, to answer those questions properly. I thought that was a pretty creative one as well. So this is in sales automation. So it's a lot of use cases here. The most basic one is please write an email to my customer with an answer to the question they ask, which often might be set up a meeting, let's say. But an innovative one here that I like as well is when that sales rep is having a conversation with a customer, they may need information at their fingertips. Let's say the customer asks them a question about a product, well, we can use generative AI to help us. So this is where embeddings models come in. So there's a certain subset of generative AI, which is about being able to figure out similarity.
So it's not just searching based upon strings, it's actually knowing that a canine and a dog are actually the same thing and that a German Shepherd is a type of dog. So if somebody's asking you about dogs and you have something about German Shepherds, that's probably the closest thing, even though you would never find that with a string match. So this is of course in a customer decision hub. The place where we've started here is on treatment generation. So those familiar with CDH know that CDH lives on content. It needs lots of different ways of saying the same thing. It needs lots of different things that it could talk to your customers about. And often what we see is the bottleneck to getting a lot of value out of CDH is actually having the creative abilities to create ... come up with all those different treatments. We call them treatments. So this is something that GPT is great at.
So what we do here is first we look through the system and analyze it, find the places where there'll be the most impact to adding more content. So for example, we might realize that there's an action for a gold credit card. That only has one treatment for it, right? The models aren't going to be able to experiment with different ways of presenting that. So that might be a very high priority one. And what it will do is it will go out, ask GPT to generate three or four variants, and bring them forward ... and this is going back to what Christian was saying. Back to the human to review them and make sure that they're in line with your company brand and values. And then of course you can use the tool. You can give it extra hints for how you might want to generate those treatments.
There are some ... so Rob mentioned them in his keynote. We've built in Cialdini's Principles of Persuasion and we'll auto-generate treatments using those different principles. So one of them might be authority, which is, "You should buy this product." Social proof would be, "Did you know that all your friends have this product?" Weave that in to the treatments. So for operations, this is really getting insights into your case data, but being able to ask questions in natural language and just get the answer. What I think is most exciting about this is not having to understand the data model. Again, you could ask the question and it will figure out what fields in your schema you would've needed to know about in order to slice and dice the data to come up with your answer.
This one is about explaining how decisions are made. So this particular example is an example for process AI, which is using Pega's decisioning technology for ... the most common use case would be streamlining a step in a case. So in an insurance approval, let's say you know this case is probably going to end up being approved, you could streamline that and do straight through processing. But the person who's auditing that later might want to know why. What was the thing about this particular case that made you decide to streamline it? And so this will explain in natural language the rationale behind the decision. This is something, a pattern, that you'll see a lot also in CDH is being able to ... we have another use case that's not in this slides. We call it chat with your decision results. So you can actually simulate a customer decision.
It'll come up with, "Here are the 30 things we could have said. Here are the 27 that got filtered out, here are the three that passed through." And you could ask it a question like, "What was the propensity for the highest action that got suppressed?" That can give you an idea of what you may be cutting off your customers from getting to see if you had less restrictive rules. This is an interesting one. This is an application that we have for doing financial crimes reporting. And so the scenario here is an investigator has figured out that there's a certain series of transactions that are most likely fraudulent and they have to write up a document explaining it so they could submit it to the committee that's in charge of tracking these things down. They have to be written in a certain way, and this is another a great thing that generative AI can do for you. So with that, I'm going to pass it over to Peter. We're going to talk a little bit about the future.
Peter van der Putten:
Awesome. Thank you very much, Shoel. It's a quite impressive range of capabilities that the product and the engineering teams have- that put together. Sorry to have put you into this mess.
Shoel Perelman:
I love it. Every minute.
Peter van der Putten:
Yeah, no, but it's fun. So let's talk a little bit about the future. So we've launched it here at PegaWorld. These capabilities will be available in Pega 2023. As a preview feature, we do still need to determine how exactly the licensing and the pricing is going to work. That probably also depends on the use case, but that will come with Pega 2024. In Pega 2023, you can use these capabilities as preview. You would bring your own key. So your OpenAI key or some other generative AI provider, at least for the 2023 version. That also makes it actually for you internally also easier in terms of-
Shoel Perelman:
And just to-
Peter van der Putten:
... not just the pricing.
Shoel Perelman:
One more addendum on that. So we will be adding bring your own key, but to make it really easy to get started, on Pega Cloud, this will just work. So if you're on Pega Cloud, that will be the quickest way of being able to make use of this first.
Peter van der Putten:
what I wanted to say that it also covers some of the legal points around liability and stuff like that. Yeah, so that's a little bit the rollout plan. And yeah, you've seen that we were able to ... We call it 20 boosters, but if you walk into the tech pavilion, you probably see a lot more than just 20 boosters out there. So we're really developing this out very, very quickly. Now, in terms of what's coming up next, maybe from a slightly more research perspective, we've been usually using the term generative AI, GPT, et cetera. But let's be clear, we are very much for choice of your generative AI provider, that's very much our strategy. So that means not just OpenAI, but we're working very closely with AWS. So actually when AWS launched their generative AI offering, they only mentioned two vendors as early adopters of this technology, which is AWS Bedrock and their future Titan models.
So Pega was only the one of two vendors that was mentioned by AWS. But likewise, we are also a trusted tester for Google generative AI. So we have access to particular AI models, et cetera, foundation models, that are not out yet in preview for Google users. We can already test them out. And we're really working very closely with the Google team as well in the Genitive AI space, plus all the work we're doing on these open source models where ... for the particular use cases that are maybe very, very private where you don't want to call out to a third party. Yeah, so the other thing that's interesting, maybe more from a researcher point of view, is giving these large language models tools, giving them sensors. So with sensors, I mean, also access to do a search or on the internet, or maybe it could be a private service in your environment to retrieve customer data.
And so the language models can then actually sense the environment more. Maybe you also can give them actual tools where they can actually take actions within constraints, the things that you want to allow them to do automatically. And then ultimately the exciting part is when generative AI is also used to generate plans on what to do next. So in that sense, the generative AI, it's not just making suggestions, it's things like, oh, I have a claim coming in. Maybe let's first retrieve some more information about this customer in the back end. And then it has that information as well. Because it's a complex claim, we need to route it to a different department, so ... then this autonomous angle really comes into play. Potentially very powerful but it also needs to be governed really, really well. And indeed, like I said, i have more specific to the product. Where we're going is to make it very easy to support a variety of these different types of large language model providers or generative AI providers in general.
I think the key point is that we have a future-proof architecture. If you have a central API where you can say, oh, I'm calling generative AI with a particular request, and I get output returned. And one day you maybe have GPT-4 behind it. The next day, you say, let's switch that to GPT-3.5 because of cost. And then you say, oh, we enter into a strategic agreement with Google or AWS, so we're switching to either Google or AWS. So you can switch that in the back end without affecting any of your boosters and use cases because they just continue to work. But we want to build it out further. It also gives us a central place to build out the PAI filtering and things like that. And then we will continue to build out these use cases, as you could see, beyond the 20 use cases that we already have built. So with that, I want to say ... I want to encourage you just to try it out in Pega Infinity 23 as it comes out in Q3, and also to explore those use cases in the tech pavilion. And I would like to open the floor for questions.
Yeah, there's microphones in the back.
Speaker 5:
Hi, my name is Abhishek. Thank you so much for the great presentation. And I'm pretty sure this is just a tip of the iceberg. I mean, there's a lot for us to learn being a total new technology. My question is about ... generative AI is a very new technology for most of the organization. They have never explored how they can use this in their day-to-day applications. And there are a lot of regulatory issues in those organizations. Not everyone is really open to use them right away. So Pega has introduced this with upcoming 23. What would you suggest to this organization? How quickly and how confidently they can start using it. Because until they open up, they won't be able to use these abilities at all.
Peter van der Putten:
Yeah. So I think what you see here in our approach, we particularly try to address some of those issues. One, by having that central architecture that gives you a central place to do two things. One is to control things like making sure that there's no PII data, for example, in the calls that you make out to generative AI. But the other one, like I explained, the generative AI ecosystem in the back end is very quickly developing. But by having that central point where you can manage what kind of generative AI you want to use, you can control that ... well, not control that risk. You can have your use cases and you can simply ... if new models come out that perform better and you just switch them out in the back end.
And the other part, we've spoken here about these boosters, these capabilities that we've built, but we've been quite selective in terms of where do we want to build ... what kind of use cases do we want to build first? Shoel, you were talking about over 300 IDs that were submitted but actually also prototyped and built. But still whittled it down and said like, hey, what are the use cases that primarily have a human-in-the-loop? What are use cases where you minimize the chances of leaking proprietary data or personal data to the generative AI service? What are the use cases that will give you the biggest return? So we have been quite deliberate in choosing particular use cases that would maximize impact and minimize risk in that sense.
Shoel Perelman:
But I would say take these and pitch them because these are the ones that I think meet all those criteria.
Speaker 5:
Makes sense. Thank you so much.
Shoel Perelman:
You're welcome.
Speaker 6:
To keep the questions baited here, human-in-the-loop, what's your basic guidance on when it's okay no human in the loop, when it's heavy you really need them in the loop all the time like watchdogs and medium case in between? What are you seeing there is evolving? And the last, just to chum the water a little bit more, cracks me up from the marketing standpoint, this almost acts like do we want legal in the loop? Do we want compliance in the loop? Do we want security in the loop? To which the marketer's answer is, "No, no, no. None of them in the loop."
Peter van der Putten:
Great question. So first and foremost, it's not ... there might be use cases where ... that are more direct to the customer, but you have identified that the risk of hallucination, whatever, is acceptable. But it's more like a general point of view that it's good to start and look at what use cases are human-in-the-loop because you minimize your risk. You want all your initial pilots to be very successful. So it's not binary. But it's just like where most ... It's good to go for the high return, low risk use cases first. Also, in the loop, it sounds like always in the loop.
To take that marketing example, when we generate new treatments, let's say we have a platinum card and there's three ways how you could describe how you could advertise the treatment to your customers, then the human in the loop is the marketer who says like, "Oh, I like this treatment, not that treatment. Here I'm making some final changes." Then pushes the button and says, "Now these treatments are available." But then you're also done. Then, in this example, the customer decision hub will continue to decide on what is the next-best-action and learn against these treatments and things like that. And so human-in-the-loop, that could also be primarily, let's say, at the design time process and then run time ... yeah, everything works. So it also doesn't need to be too obstructive in that sense to have the human in the loop.
Christian Guttmann:
Yeah, we come quite close also to this concept. You're probably familiar with human-on-the-loop, human-outside-the-loop. So we are probably not going humans outside the loop, but we are getting quite close to humans on the loop. Meaning there's this monitoring and very minimal interactions to accept what our GenAI is producing. So to make that interaction very easy and very intuitive. And then I would say, I think the general guideline as to how we are implementing it and when we let AI make more decisions, I mean, basically, if what's happening in the background when you have autopilot are sub steps of a bigger plan, then that would be something that could of course be done to a certain extent if it doesn't have any major consequence at that time. But that's some thoughts on that topic.
Peter van der Putten:
Okay.
Christian Guttmann:
Right on time.
Peter van der Putten:
Thank you very much. With that, we are exactly at time. If you have any further questions, we will stay around for a bit. So feel free to come up and ask us any questions. Also explore the demos in the tech pavilion and particularly ask for how GenAI is being used and just get your hands on Pega Infinity 2023 Lab Instance the moment they come out and play with the technology. I would encourage you to have a bit of fun with the technology as well. Best way to explore it. Thank you very much. Thank you.
Related Resource
Produit
Une révolution dans la conception d’applicationsOptimisez et accélérez la conception de workflows, grâce à la puissance de Pega GenAI Blueprint™. Présentez votre vision pour générer votre workflow instantanément.